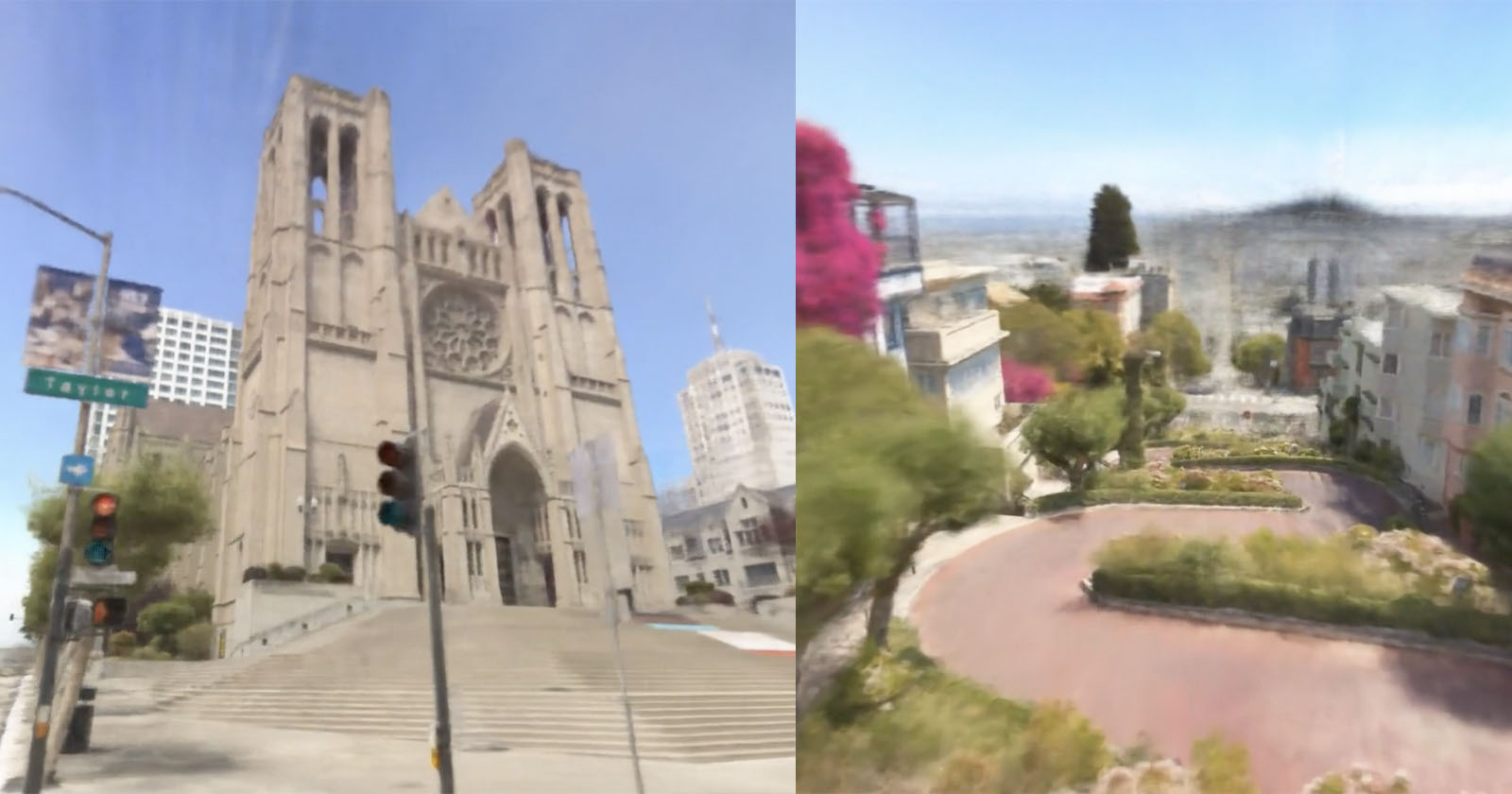
Current developments in synthetic intelligence (AI) permit 3D scenes to be generated from only a handful of photographs, however Waymo researchers pushed the thought to the extremes and have efficiently recreated a 3D mannequin of San Francisco from 2.8 million enter photographs.
Neural Radiance Fields
Neural radiance filds (NeRF) have been used to synthesize views of complicated scenes by taking a number of enter pictures and utilizing an AI to generate the gaps. A number of corporations have experimented with the know-how, most notably Google researchers — who reconstructed areas utilizing a batch of photographs from vacationers — and NVIDIA — which not too long ago debuted a neural rendering mannequin that may produce 3D scenes from a small variety of photographs.
However researchers from Waymo — previously Google’s self-driving automotive division — determined to take the idea to the following degree, as noticed by DIY Images. The group says that whereas they discovered the present purposes of NeRF attention-grabbing, they weren’t notably helpful as they solely recreated a single location.
“Current developments in neural rendering corresponding to Neural Radiance Fields have enabled photo-realistic reconstruction and novel view synthesis given a set of posed digicam pictures,” the group says in its analysis paper.”Earlier works tended to deal with small-scale and object-centric reconstruction.”
Block-NeRF
The group created what it calls Block-NeRF — a variant of Neural Radiance Fields that may characterize massive environments — to make large-scale scene reconstructions by utilizing a number of NeRFs and mixing the renderings collectively. The researchers had been in a position to make use of an enormous quantity of visible knowledge that was captured by Waymo’s self-driving vehicles which have been examined via San Francisco.
“We constructed a grid of Block-NeRFs from 2.8 million pictures to create the most important neural scene illustration to this point, able to rendering a complete neighborhood of San Francisco,” the group says.
The researchers took the Alamo neighborhood — which is roughly a half sq. kilometer in dimension — and rendered it in 3D utilizing 35 Block-NeRFs, one for every block. To create the render, Waymo researchers used 2.8 million photographs that had been captured over a three-month interval. On the analysis web site, the group recreated extra than simply the Alamo Sq. block and has 3D recreations of Lombard Avenue, the Bay Bridge and the Embarcadero space, the Moscone Heart, and the downtown space. Every of those renders took its personal set of hundreds of thousands of pictures to create.
Constructing a 3D surroundings this massive just isn’t with out its challenges. The presence of transferring objects like vehicles and folks, limitations on the capability of particular person NeRF fashions, and points with reminiscence and computing constraints makes such a digital recreation tough. The researchers tackled these points by breaking apart the massive environments into individually educated “blocks” (therefore the identify Block-NeRF) that are then dynamically mixed. In keeping with the researchers, this methodology supplies sufficient flexibility and scalability to allow the recreation of enormous environments.
The Waymo researchers say that reconstructing large-scale environments allows a number of essential use-cases, particularly for autonomous driving and aerial surveying.
A bonus of the Block-NeRF strategy is that when the 3D surroundings has been created, the digital house just isn’t confined to the trail that the Waymo self-driving vehicles traversed and will be totally explored from any angle.
“One instance is mapping, the place a high-fidelity map of the whole working area is created to behave as a robust prior for quite a lot of issues, together with robotic localization, navigation, and collision avoidance,” the researchers clarify.
The total analysis paper and a number of other examples of the Block-NeRF’s capabilities will be discovered on the Waymo analysis web site.