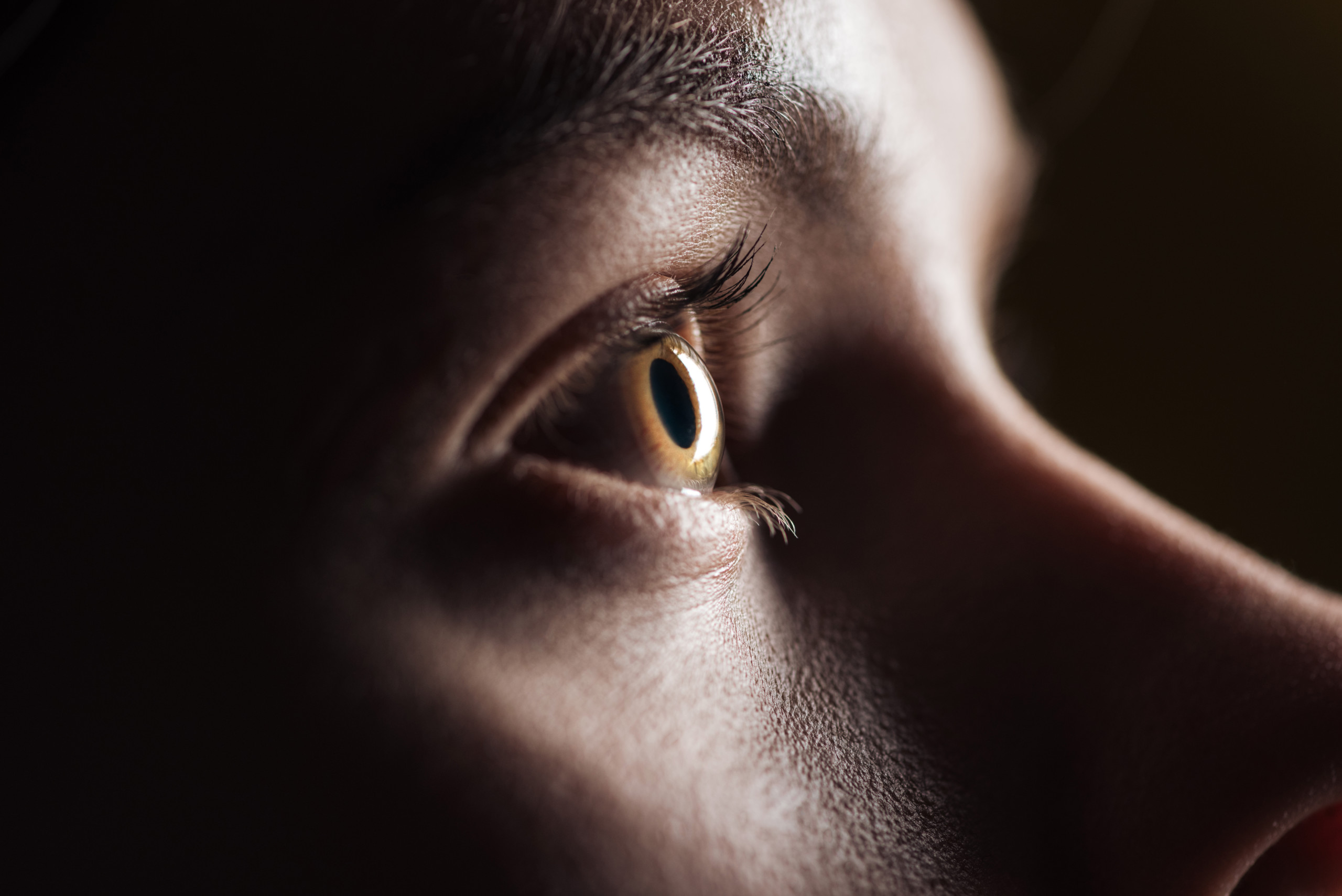
A workforce of scientists has created an algorithm that may label objects in {a photograph} with single-pixel accuracy with out human supervision.
Referred to as STEGO, it’s a joint mission from MIT’s CSAIL, Microsoft, and Cornell College. The workforce hopes they’ve solved one of many hardest duties in pc imaginative and prescient: to assign a label to each pixel on the earth, with out human supervision.
Pc imaginative and prescient is a discipline of synthetic intelligence (AI) that allows computer systems to derive significant info from digital photographs.
STEGO learns one thing known as “semantic segmentation,” which is the method of assigning a label to each pixel in a picture. It’s an essential ability for as we speak’s computer-vision system as a result of as photographers know, photographs will be cluttered with objects.
Usually creating coaching information for computer systems to learn a picture includes people drawing packing containers round particular objects inside a picture. For instance, drawing a field round a cat in a discipline of grass and labeling what’s contained in the field “cat.”
The semantic segmentation approach will label each pixel that makes up the cat, and received’t get any grass blended up. In Photoshop phrases, it’s like utilizing the Object Choice software moderately than the Rectangular Marquee software.
The issue with the human approach is that the system calls for 1000’s, if not tons of of 1000’s, of labeled photographs with which to coach the algorithm. A single 256×256-pixel picture is made up of 65,536 particular person pixels, and making an attempt to label each pixel from 100,000 photographs borders on the absurd.
Seeing The World
Nonetheless, rising applied sciences are requiring machines to have the ability to learn the world round them for issues similar to self-driving automobiles and medical diagnostics. People additionally need cameras to higher perceive the images it’s taking.
Lead writer of the brand new paper about STEGO, Mark Hamilton, means that the know-how may very well be used to scan “rising domains” the place people don’t even know what the suitable objects needs to be.
“In all these conditions the place you wish to design a technique to function on the boundaries of science, you possibly can’t depend on people to determine it out earlier than machines do,” he says, talking to MIT Information.
STEGO was educated on a wide range of visible domains, from residence interiors to high-altitude aerial photographs. The brand new system doubled the efficiency of earlier semantic segmentation schemes, intently aligning with what people judged the objects to be.
“When utilized to driverless automotive datasets, STEGO efficiently segmented out roads, individuals, and road indicators with a lot increased decision and granularity than earlier methods. On photographs from area, the system broke down each single sq. foot of the floor of the Earth into roads, vegetation, and buildings,” writes the MIT CSAIL workforce.
The Algorithm Can Nonetheless Be Tripped Up
STEGO nonetheless struggled to differentiate between foodstuffs like grits and pasta. It was additionally confused by odd photographs — similar to one in every of a banana sitting on a cellphone receiver and the receiver was labeled “foodstuff,” as an alternative of “uncooked materials.”
Regardless of the machine nonetheless grappling with what’s a banana and what isn’t, the algorithm represents the “benchmark for progress in picture understanding,” in response to Andrea Vedaldi of Oxford College.
“This analysis offers maybe essentially the most direct and efficient demonstration of this progress on unsupervised segmentation.”
Picture credit: Header picture licensed through Depositphotos.